



An introduction to Alignment
An introduction to Alignment
An introduction to Alignment
July 2023
A New Era of AI Risk
A New Era of AI Risk
In the past few years, artificial intelligence capabilities have dramatically increased in broad domains like playing games and generating text/code, images, and videos. Most of these latest advances have come from “foundation models,” large neural networks trained on massive amounts of data. These models continue to demonstrate new and more powerful capabilities the more model size, data, and compute increase.
In the past few years, artificial intelligence capabilities have dramatically increased in broad domains like playing games and generating text/code, images, and videos. Most of these latest advances have come from “foundation models,” large neural networks trained on massive amounts of data. These models continue to demonstrate new and more powerful capabilities the more model size, data, and compute increase.
As AI models increase in capabilities and complexity, they pose greater risk to individuals and society. Some of the risks are from misuse by bad actors, such as the spread of disinformation, government censorship or surveillance, or election manipulation. But other risks from models exist even without misuse, such as fundamental biases, generation of untruthful and misleading information, or physical risks from applied AI such as from self-driving cars.
As AI models increase in capabilities and complexity, they pose greater risk to individuals and society. Some of the risks are from misuse by bad actors, such as the spread of disinformation, government censorship or surveillance, or election manipulation. But other risks from models exist even without misuse, such as fundamental biases, generation of untruthful and misleading information, or physical risks from applied AI such as from self-driving cars.
Nevertheless, the promise of even more powerful capabilities has fueled a race among major tech companies to develop transformatively powerful AI systems. Many organizations have as their stated goal the creation of Artificial General Intelligence (AGI), systems that could be able to perform all intellectual tasks that humans can do.
Nevertheless, the promise of even more powerful capabilities has fueled a race among major tech companies to develop transformatively powerful AI systems. Many organizations have as their stated goal the creation of Artificial General Intelligence (AGI), systems that could be able to perform all intellectual tasks that humans can do.
Most AI Safety techniques being developed for today’s AI systems will not scale as AI becomes increasingly powerful. As laboratories get closer to developing AGI, AI Safety experts need to contend with a different set of potential risks, including catastrophic damage caused by transformatively powerful systems.
Most AI Safety techniques being developed for today’s AI systems will not scale as AI becomes increasingly powerful. As laboratories get closer to developing AGI, AI Safety experts need to contend with a different set of potential risks, including catastrophic damage caused by transformatively powerful systems.
We’re running out of time to create these guardrails, and an increasing number of experts are sounding the alarm.
We’re running out of time to create these guardrails, and an increasing number of experts are sounding the alarm.
“Mitigating the risk of extinction from AI should be a global priority alongside other societal-scale risks such as pandemics and nuclear war."
“Mitigating the risk of extinction from AI should be a global priority alongside other societal-scale risks such as pandemics and nuclear war."
“Mitigating the risk of extinction from AI should be a global priority alongside other societal-scale risks such as pandemics and nuclear war."
Signed by Sam Altman, Demis Hassabis, Dario Amodei
Signed by Sam Altman, Demis Hassabis, Dario Amodei
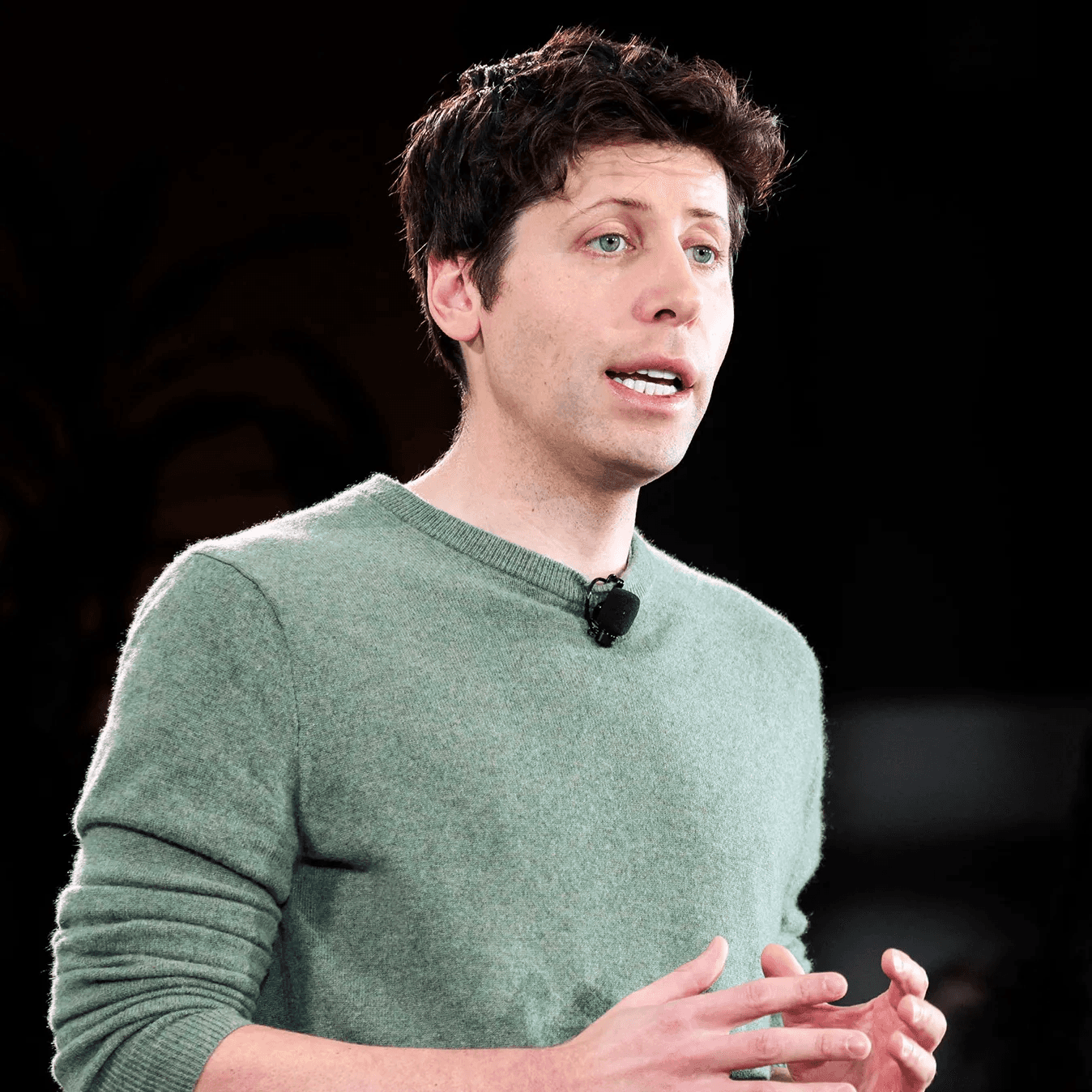

edition.cnn.com
Exclusive: 42% of CEOs say AI could destroy humanity in five to ten years.
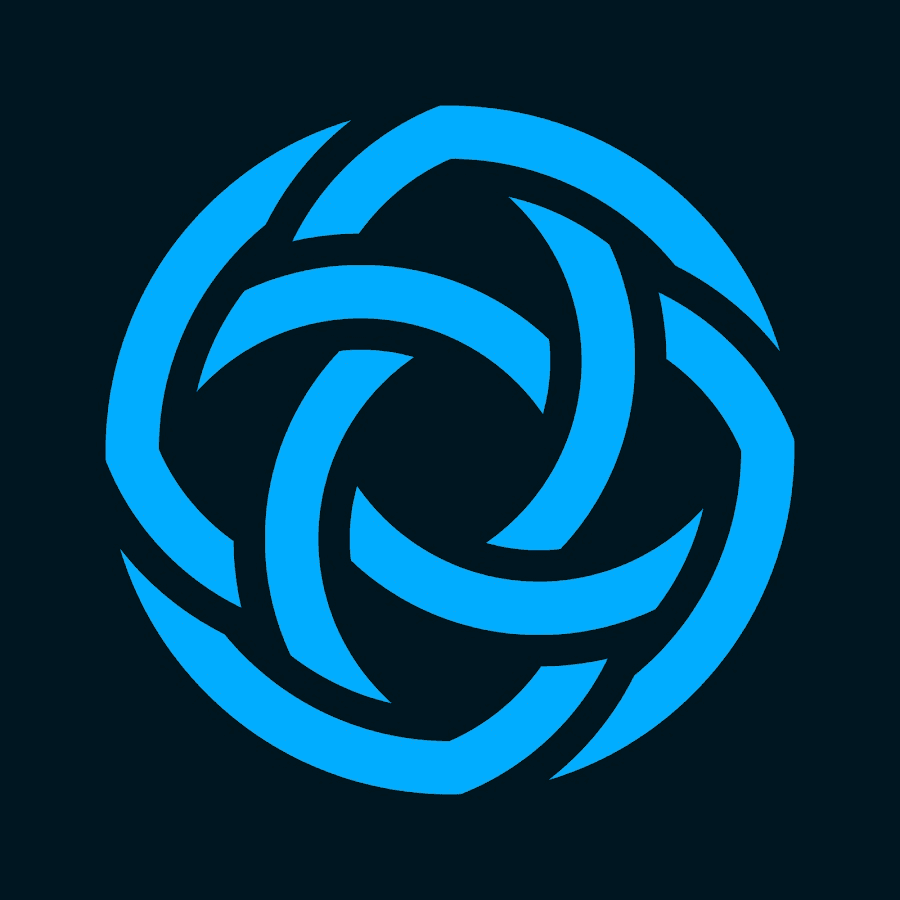
Center for AI Saftey
“Mitigating the risk of extinction from AI should be a global priority alongside other societal-scale risks such as pandemics and nuclear war.

BBC.com
AI ‘godfather’ Yoshua Bengio feels ‘lost’ over life’s work


edition.cnn.com
Exclusive: 42% of CEOs say AI could destroy humanity in five to ten years.
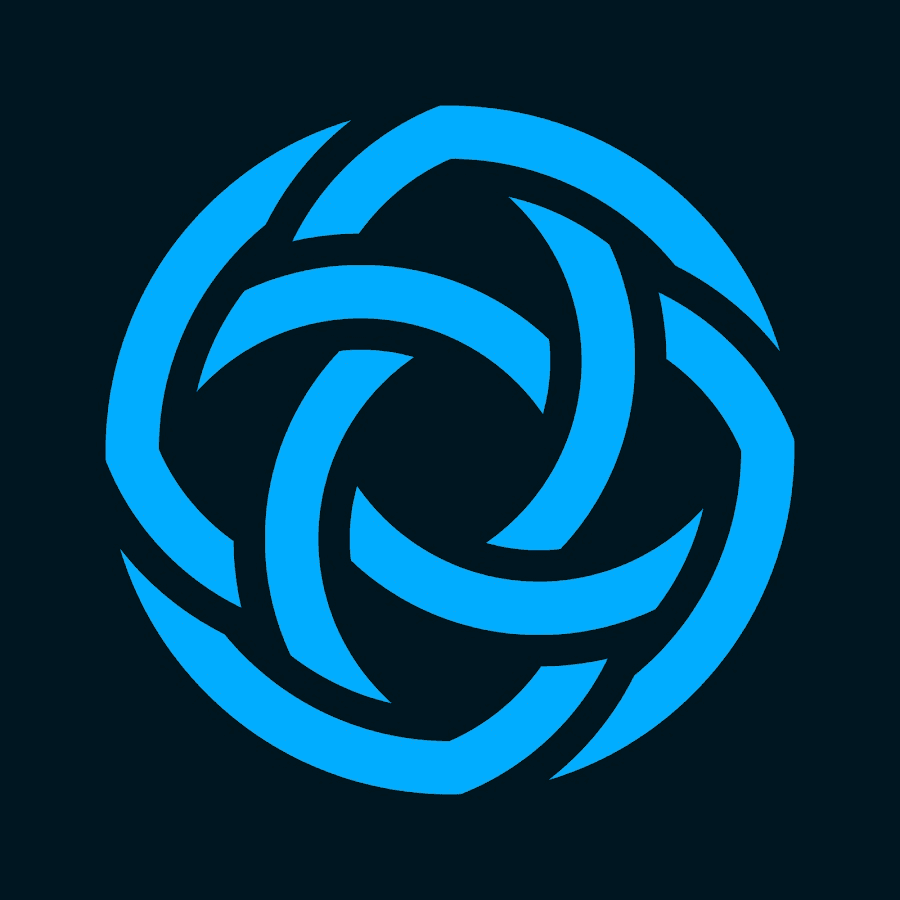
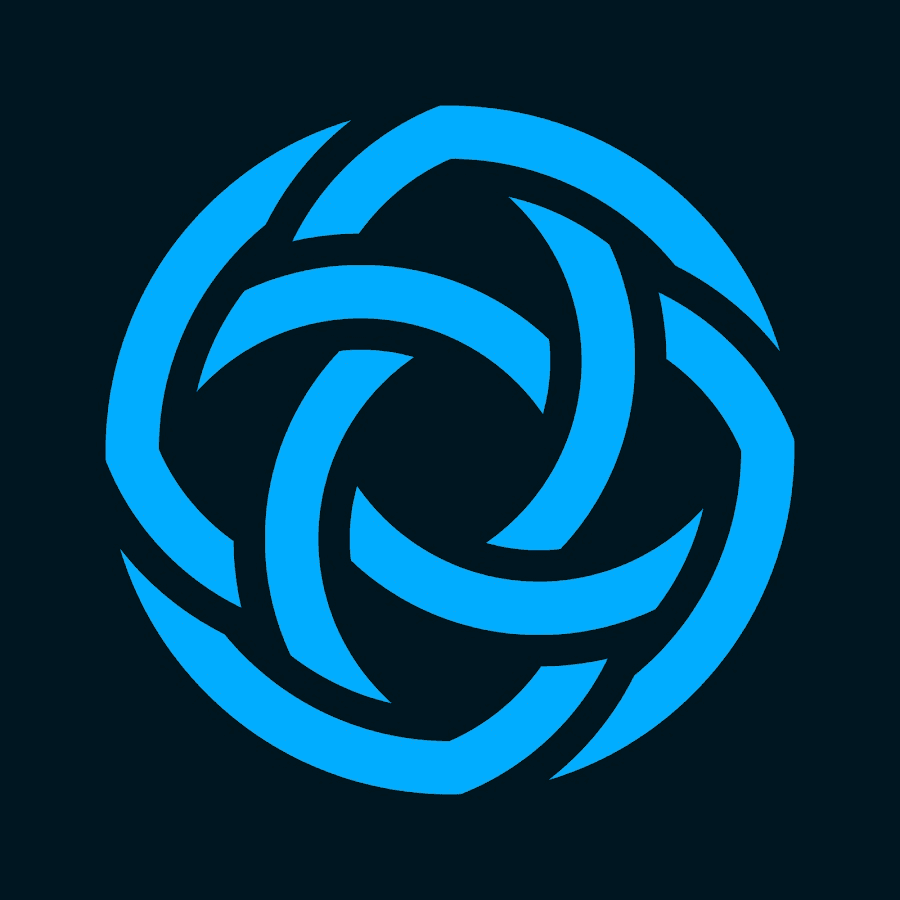
Center for AI Saftey
“ Mitigating the risk of extinction from AI should be a global priority alongside other societal-scale risks such as pandemics and nuclear war.


BBC.com
AI ‘godfather’ Yoshua Bengio feels ‘lost’ over life’s work
AI Alignment
AI Alignment
There are a number of researchers around the world working on AI safety for existential-risk level threats. This subfield of AI Safety is called “AI Alignment” and is generally concerned with the problem of directing Artificial Intelligence towards desired outcomes, particularly as the responsibilities left to AIs and the capabilities of AIs increase.
There are a number of researchers around the world working on AI safety for existential-risk level threats. This subfield of AI Safety is called “AI Alignment” and is generally concerned with the problem of directing Artificial Intelligence towards desired outcomes, particularly as the responsibilities left to AIs and the capabilities of AIs increase.
Alignment fundamentally is a field that faces extremely difficult problems and incredibly high demands for safety, that are in many ways unlike most mainstream research in AI safety.
Alignment fundamentally is a field that faces extremely difficult problems and incredibly high demands for safety, that are in many ways unlike most mainstream research in AI safety.
Because alignment solutions must be robust to scaling, researchers cannot assume some reasonable limit on the capabilities and responsibilities of AIs, leading to the failure of many bounded solutions.
Because of the potentially massive consequences of malfunction, researchers cannot iterate on powerful AIs and catch alignment problems as they crop up.
Because AIs act in the world and react to our interventions on them, researchers cannot treat alignment as a static problem of natural science.
Because alignment solutions must be robust to scaling, researchers cannot assume some reasonable limit on the capabilities and responsibilities of AIs, leading to the failure of many bounded solutions.
Because of the potentially massive consequences of malfunction, researchers cannot iterate on powerful AIs and catch alignment problems as they crop up.
Because AIs act in the world and react to our interventions on them, researchers cannot treat alignment as a static problem of natural science.
All these subtleties require a complete reworking of methods to tackle alignment, and the risks entailed by the loss of control of rapidly improving AI.
All these subtleties require a complete reworking of methods to tackle alignment, and the risks entailed by the loss of control of rapidly improving AI.
As it stands, the world is not in a good position to make AI Alignment go well. AI capabilities are advancing far faster than safety research can keep up. While there are some positive trends - for example, existential risk from AI is now taken seriously as a mainstream concern - there is still a massive amount of work to do.
As it stands, the world is not in a good position to make AI Alignment go well. AI capabilities are advancing far faster than safety research can keep up. While there are some positive trends - for example, existential risk from AI is now taken seriously as a mainstream concern - there is still a massive amount of work to do.
At Conjecture, we believe the best approach to the “Alignment Problem” right now is first to buy time, while also working on the more limited subproblem of how to bound AI to sub-superintelligent levels. We are not equipped as a society to handle the profound risks from advanced artificial intelligence, and we need more time to figure out what we’re going to do. Scaling larger and larger models with the hopes of building superintelligence is reckless, and needs to stop.
At Conjecture, we believe the best approach to the “Alignment Problem” right now is first to buy time, while also working on the more limited subproblem of how to bound AI to sub-superintelligent levels. We are not equipped as a society to handle the profound risks from advanced artificial intelligence, and we need more time to figure out what we’re going to do. Scaling larger and larger models with the hopes of building superintelligence is reckless, and needs to stop.
At Conjecture, we’re working on an alternative approach called Cognitive Emulation to still use AI, while circumventing the risks associated with scaling.
At Conjecture, we’re working on an alternative approach called Cognitive Emulation to still use AI, while circumventing the risks associated with scaling.
Related Articles
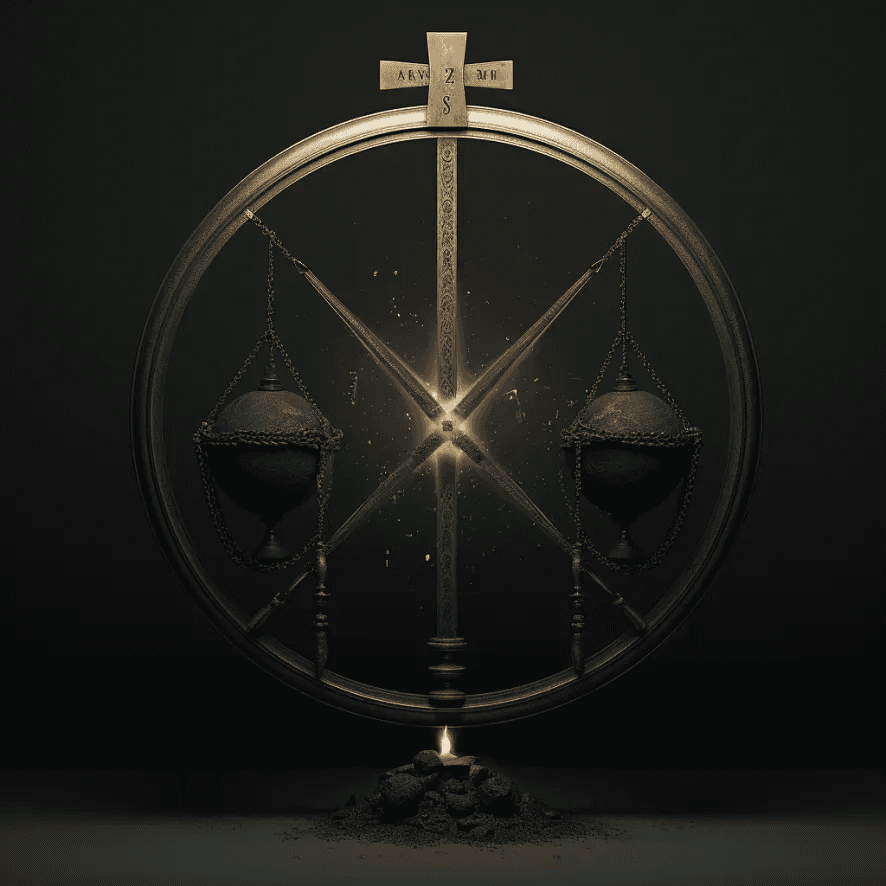
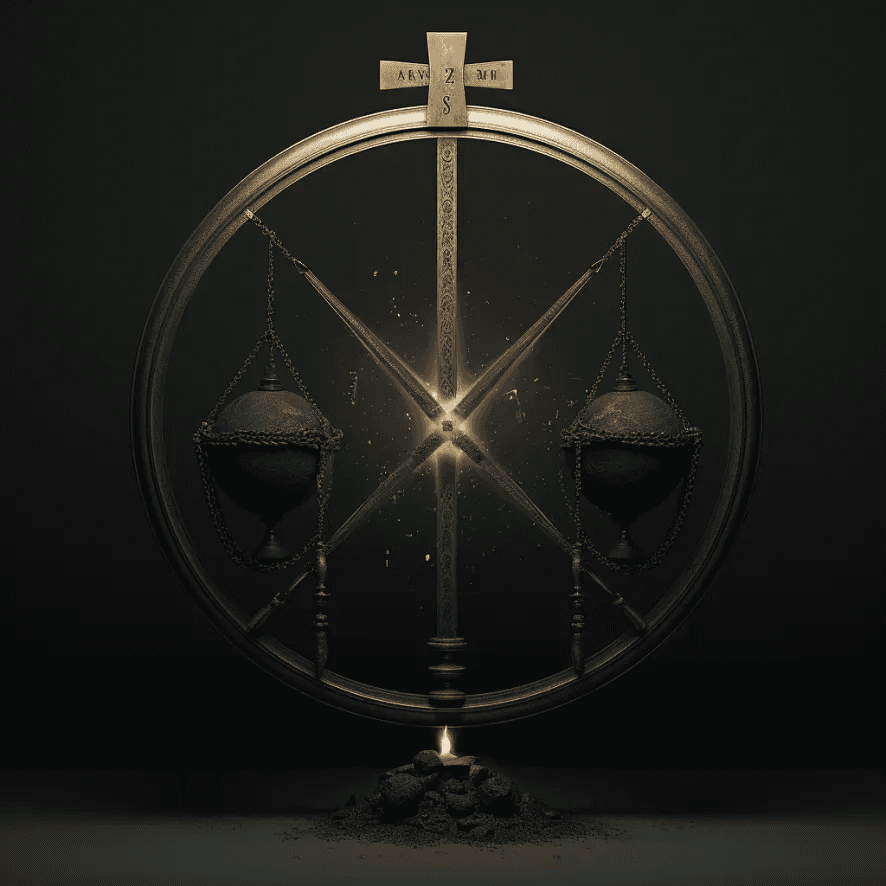
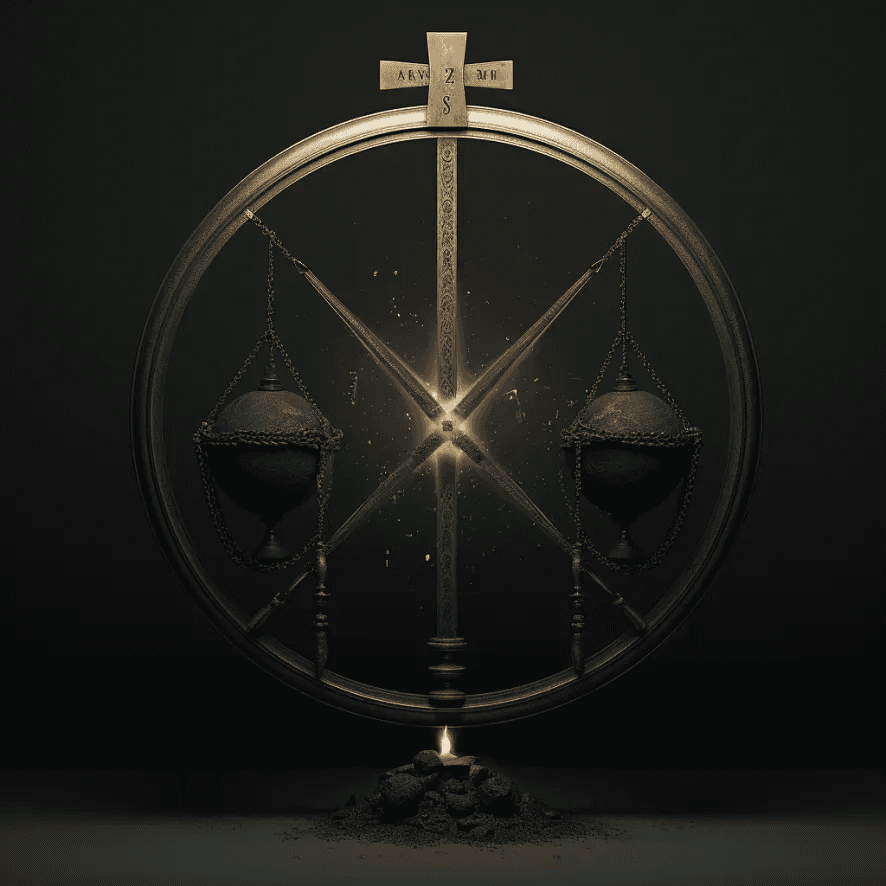
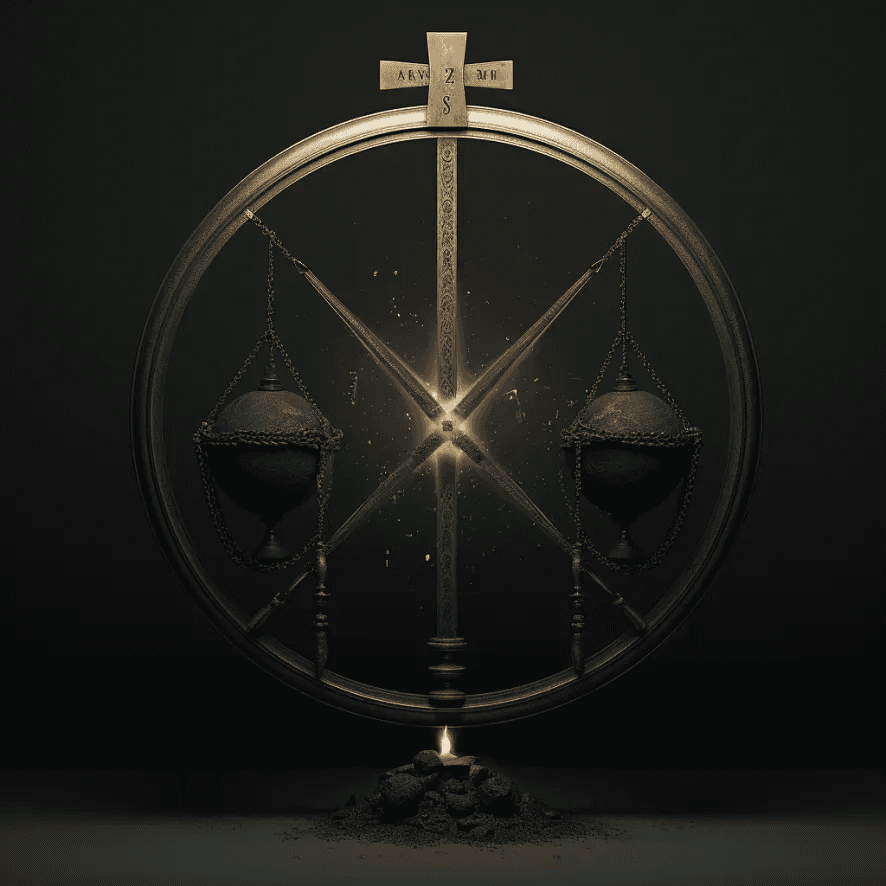
Feb 24, 2024
Christiano (ARC) and GA (Conjecture) Discuss Alignment Cruxes
The following are the summary and transcript of a discussion between Paul Christiano (ARC) and Gabriel Alfour, hereafter GA (Conjecture), which took place on December 11, 2022 on Slack. It was held as part of a series of discussions between Conjecture and people from other organizations in the AGI and alignment field. See our retrospective on the Discussions for more information about the project and the format.
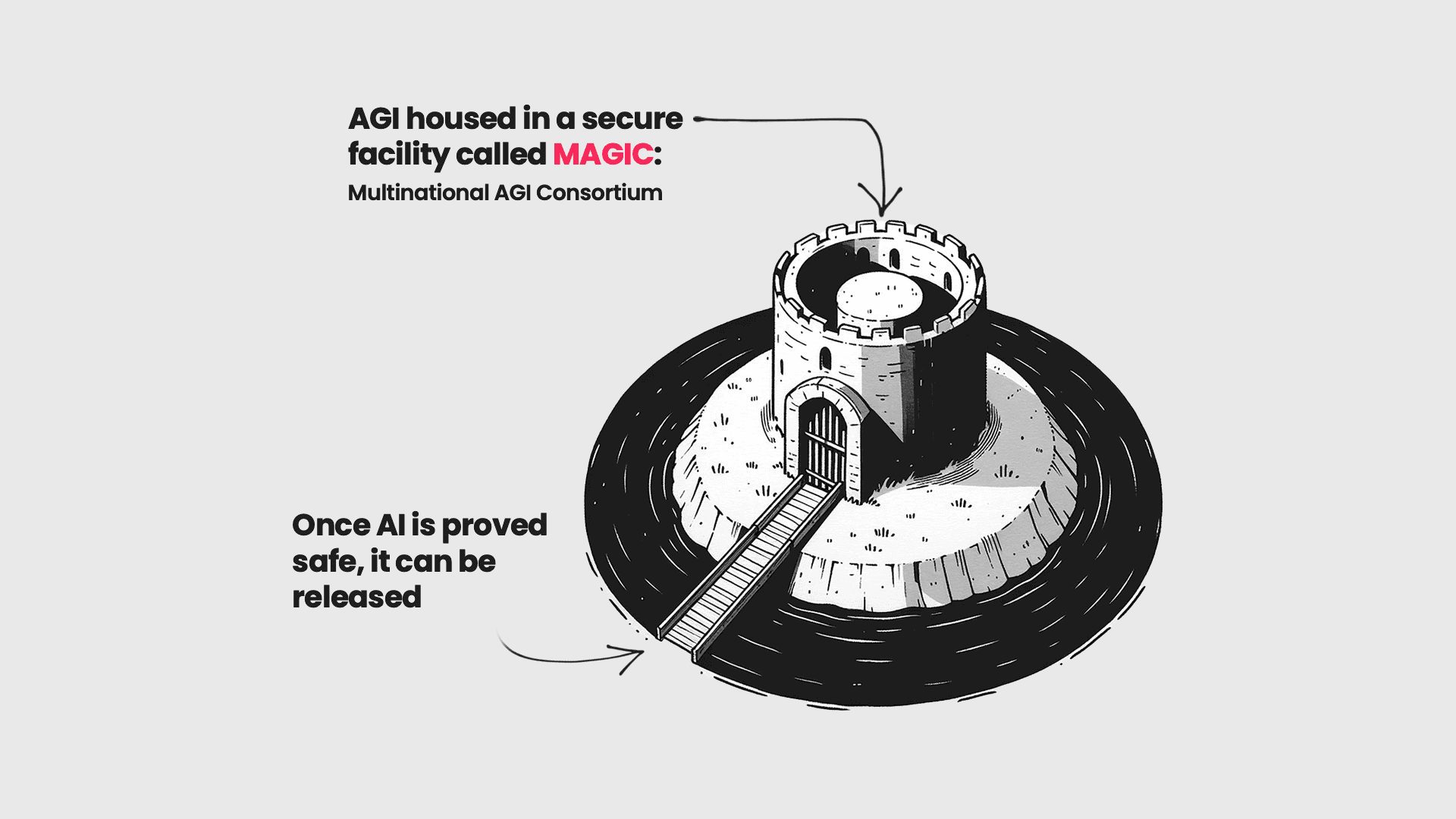
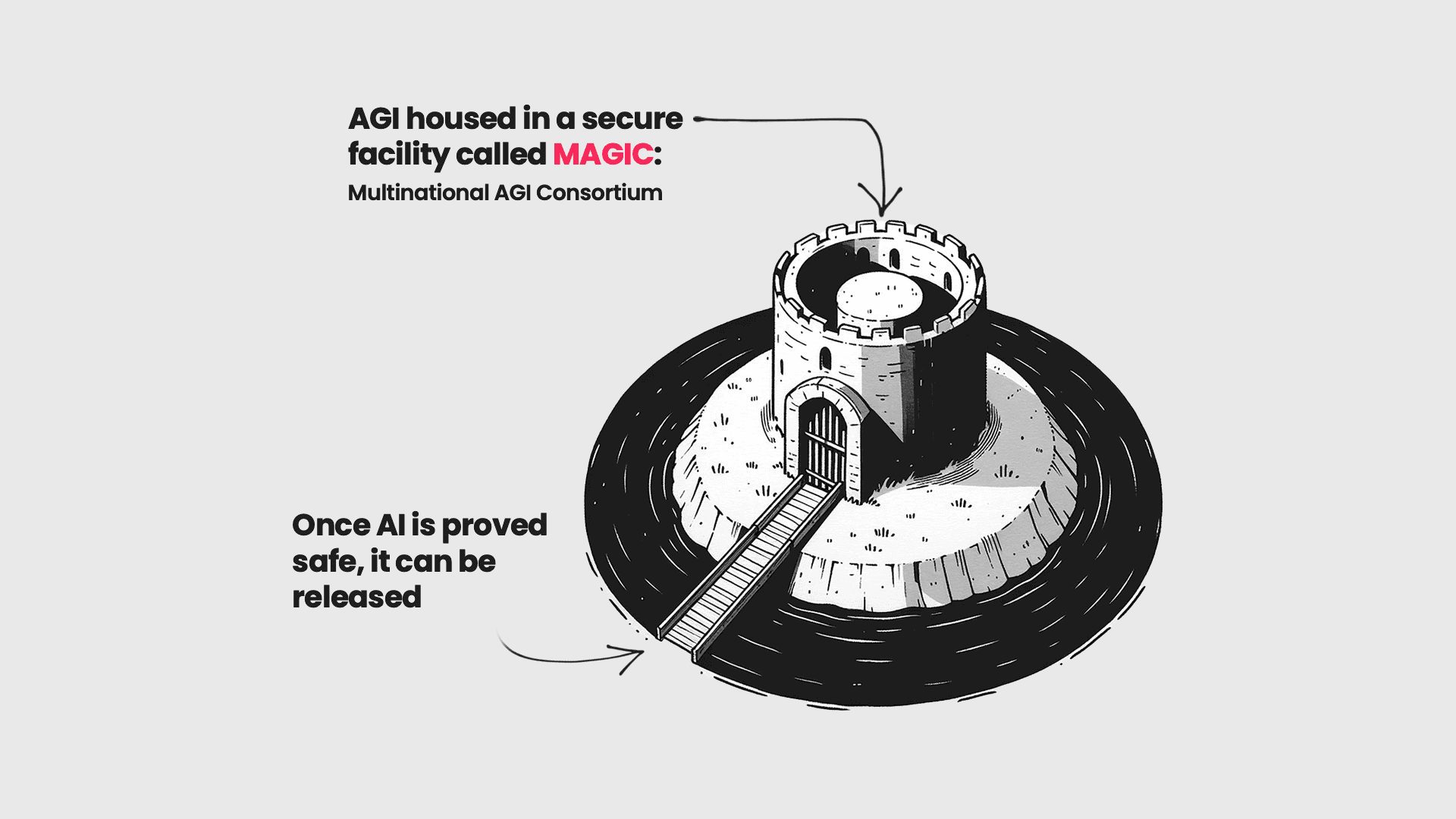
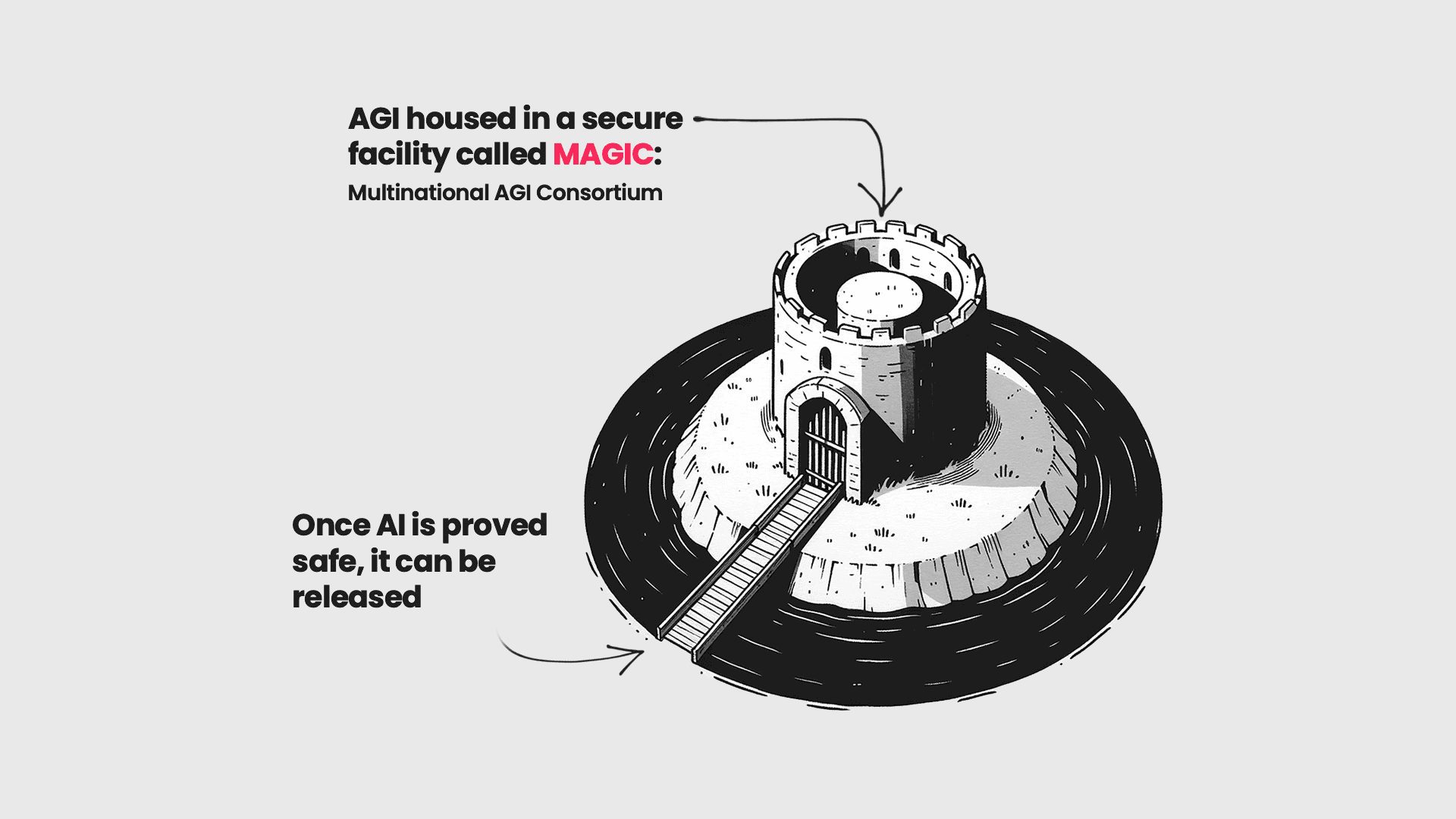
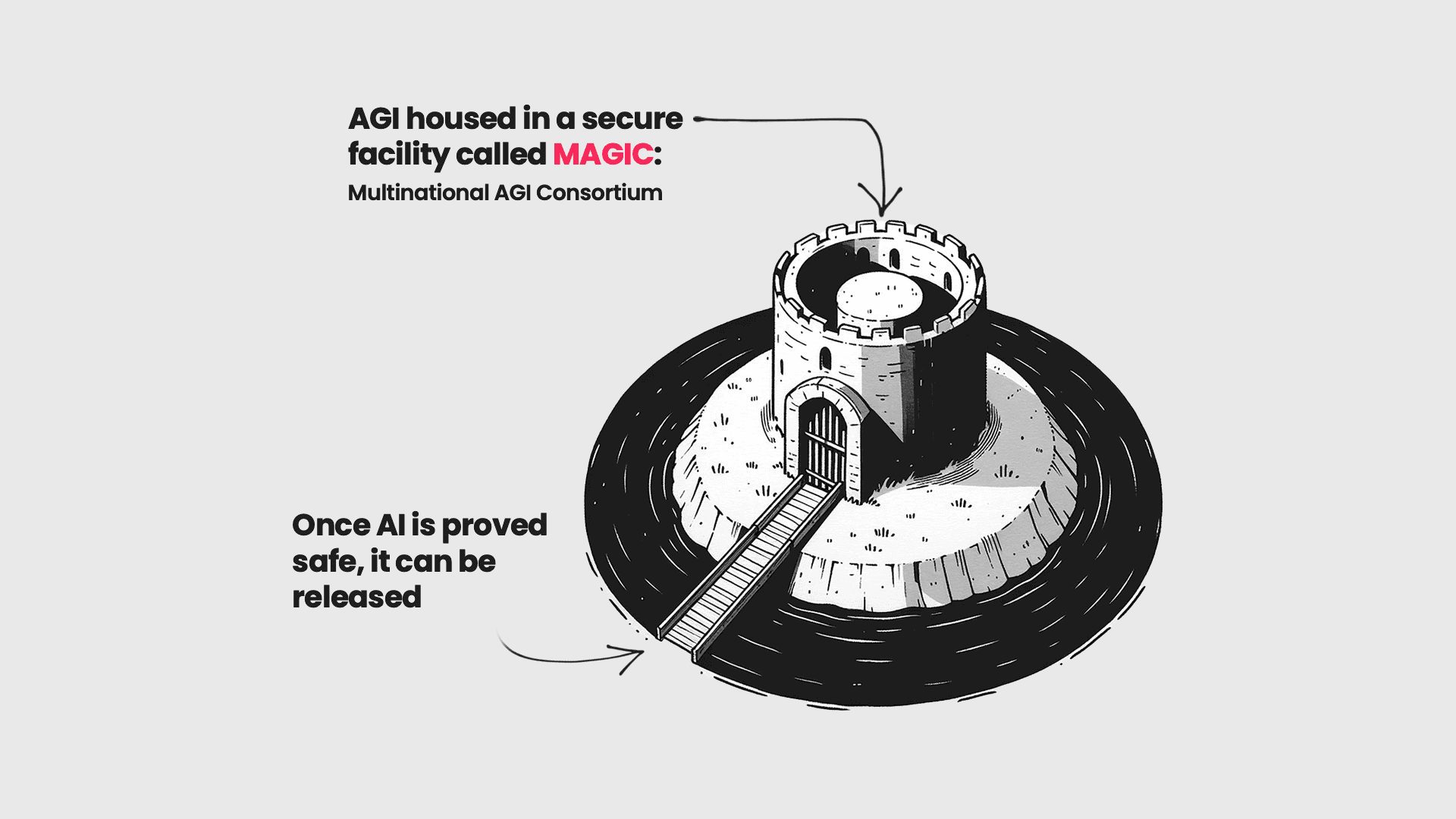
Oct 13, 2023
Multinational AGI Consortium (MAGIC): A Proposal for International Coordination on AI
This paper proposes a Multinational Artificial General Intelligence Consortium (MAGIC) to mitigate existential risks from advanced artificial intelligence (AI). MAGIC would be the only institution in the world permitted to develop advanced AI, enforced through a global moratorium by its signatory members on all other advanced AI development.




Oct 12, 2023
unRLHF - Efficiently undoing LLM safeguards
Produced as part of the SERI ML Alignment Theory Scholars Program - Summer 2023 Cohort, under the mentorship of Jeffrey Ladish. I'm grateful to Palisade Research for their support throughout this project.
Sign up to receive our newsletter and
updates on products and services.
Sign up to receive our newsletter and updates on products and services.
Sign up to receive our newsletter and updates on products and services.